As technology evolves and we enable AI to make more decisions for us we need to be confident it’s leading us in the right direction. Automated systems are increasingly being for all types of use cases in HR, sales, IT, and cybersecurity to speed up business and provide better services. However, while AI-assisted answers can be incredibly helpful, it is important to ensure these systems are properly trained and have the right context to give us accurate responses and advice. We need to build trust in AI decisions. Trusting AI means understanding that there are some inherent risks involved with relying on it for critical decisions.
Trusting AI requires context
The most important factor when considering whether or not to trust AI is context:
What is the AI being asked to do?
What information does it have access to?
Is it capable of making accurate decisions based on this data?
For AI to be trusted, it must be trained properly and given access to the right data.
Without sufficient context, AI could make errors that would have otherwise been avoided. For example, if an organization’s HR department was using AI-assisted vacation time calculations, but the program didn’t understand how seniority or job title impacted these decisions, it could lead to unfair results. Similarly, if a company was relying on an automated threat assessment system but did not provide it with enough data about past threats and similar situations, then the AI would likely miss potential dangers and leave the organization exposed to risk.
For AI-assisted systems to be trusted and used reliably there needs to be sufficient training and understanding of the context. Organizations should make sure that the AI has access to the right data and is well-trained in the tasks it is being asked to perform. It is also important to remember that AI can only be as accurate and reliable as the data it has been given, so organizations must be diligent in ensuring they are providing consistent and up-to-date information.
Understanding AI limitations
Trusting AI means understanding its inherent limitations, such as its inability to think outside of what it’s been trained on or account for changes in context. As technology continues to evolve, having an understanding of these risks will be essential when making decisions about how much we rely on automated systems. With proper training and sufficient context, however, AI can be an invaluable tool for organizations of all sizes.
For example, AI can be used to automate mundane tasks such as customer service emails or accounts payable/receivable inquiries. By providing the AI with enough information about the scope of these tasks, it can quickly and accurately answer questions that would otherwise take a human employee much longer to respond to. Similarly, AI-assisted systems can process large amounts of data in seconds and allow organizations to better understand their customers’ needs and preferences.
Links and Resources
Speakers
Transcription
Scott: We’re going to talk about what it means to trust AI. So we’ve seen the generative AI models from open AI, from Google, and all the chatter about whether or not the answer is correct or the answer is incorrect, or how correct it is. We’re going to talk about really like, how do you trust it, should you trust it? And then where are you on that scale? So, Chris, you’ve been playing around with these models quite a bit. And you’ve got some examples that you’ve asked. I think ChatGPT first, right?
What did you ask it and then how did you feel afterwards?
Chris: I’m going to skip over the whole thing that we got the exoplanet picture wrong, because that’s just a one-off. And not very realistic, but in reality this actually came up with one of my neighbors. So she’s very tech savvy. And even though she’s in her seventies and she’s like, I saw on the news, there was an actual recall of the contact solution that I use, and she’s really worried because all the brands are being recalled. I’m told her, I haven’t heard of this. So I do Google search and I go to the FDA first and find that this is an off brand. I couldn’t even find somewhere to buy it. So I just googled recalls of saline solution or recalls of eyedrops. Well, I found a whole bunch, but they were from 2015 and 2017.
So if you Google, you’re going to get the context of like a date and a timestamp. And she Googled and just read the headlines. I asked if they are all under recall. Well, when you asked say ChatGPT in terms of AI, the same thing, you don’t give it a time horizon. It’s going to do the same thing. Like what are all the top hits that we found? So you’re going to see every company in the last 10 years had some recall. So of course someone was going to say, it’s all wrong, but in reality, you need to give us some context. If you said, were there any recalls in the last 90 days, it would probably do a better job if it was trained in the last 90 days. Let’s say, you picked a time period, it’s actually trained in, but we figured out that it’s going to give us the most populous answers. There is people, or there are people who’ve gone through, who try to cleanse it and not to have too much fake news.
But there’s the whole context of continuous updates and time horizons we have to think of. So I couldn’t quite get him to tell me like, for a specific timeline, were there any recalls and knew there were ones, new byproducts. But I think that’s the big thing, we have to do that gut check of can we actually get the training to match our questions? And it’s not going to be what we’ve done in the very traditional today. And the today world machine learning is trained well, but it’s trained at human speed. We literally have data scientists who scrub the data. They analyze it, they re-scrub the data for bias. They analyze it. So we’re never going to get to any velocity with this if we rely on people to do all the training.
So we need to get, the tech has to get a little smart, and honestly, it’s pretty freaking amazing. It’s getting smarter, but we need to figure out how to train the models at machine speed. And that’s maybe, it needs to understand context of things. So I think we’ll get to more trust when we get to a little more increased value of how are we actually training and get some extra context. I think that there’s always going to be some bias. We do a lot in our corporate enterprise to remove bias, but we have to figure out how do we change that in these new technologies themselves?
Scott: That’s interesting. The gut check is either before you ask the question or when you receive the answer, right? Because if you don’t prompt it correctly, like you were saying with the time horizon, hopefully it would be smart enough to say, hey, I don’t know because I wasn’t trained on that data, let’s say last 90 days or maybe if I get an answer back, well then I’m forced to gut check it. But it’s still, like you were saying, the human speed, right?
Chris: Yes. And we do that. So we can do different semantic searches and different things and it’s really easy for searches to get wide and get close. It’s hard for them to get narrow and accurate. That’s the challenge we have to solve for, don’t just give me an answer, give me the right answer and put it in some context so I have it.
John: Okay. I’d like to highlight something. Chris, you said that the whole thing about trust is about context. And it is not about fact checkers who are going to vet every answer to generative AI before you see it, because that’s entirely absurd, couldn’t be possible. But the learning that we already have had, as your example pointed out, is when Google results show up like being result of somebody else, it’s date and time stamped and the source is identified. We’re losing that when we get into content trained, that very same content trained into a model that is now answering for us. And somehow that needs to come back out. And I think that AI can actually be trained to understand that context is required for this answer, or what type of time horizon makes the answer accurate versus inaccurate. And all of this will be sorted out.
It’s a wonderful kind of academic maybe because none of us at least, none us on this podcast are trusting or putting our total faith in a ChatGPT answer any more than we would Bard or anybody else. There are dozens, there are more than a dozen generative AI startups that are doing a lot of amazing work, it’s great. But where this ends up a real fundamental problem is, none of the folks on this podcast are thinking we’re going to wait years to settle the score on how we’re going to figure out trust. You guys are all starting projects right now and trying to figure out how to put this in front of your people. So you’re going to put a spreadsheet and somehow upload into a ChatGPT prompt.
And then someone’s going to ask three weeks from now, who’s at work tomorrow. And it is going to answer incorrectly because that spreadsheet is three weeks old. And if you’re the type of organizations we’re typically used to working with, there’s ins and outs, and there’s changes. So we are going to have to actually do a couple of things here. First, AI is going to have to learn what context makes the answer understandable, because it’s not about whether it’s accurate or not. We deal with a measure of inaccuracy every time we do a Google search. Every time we go to Wikipedia, every time you ask an expert. What we’ve really done though is we’ve done a really good job of expecting the context to come with that answer. Either implicit in who we asked or how we asked, or the content itself.
The second half is when we are, especially in an enterprise context, when we are asking what were sales last month. That is an absolute must be answered by a system, not an interpretation of an AI model that’s reading a bunch of content. That is going to be wired up to a backend system, driving through an API, making a definitive. This is like, I’m not going to ask chat GPT how long does it take for me to drive to Austin tomorrow morning? I’m going to ask a GPS system that’s going to give me a realtime at that very moment look and predictions based on typical behavior along the way, road conditions along the way and realtime updates as I’m on the road. I am not about to ask a generative model that sort of thing.
But if I did want a generative model to do it, I’ve got to put that model in such a condition where I can get it to invoke those real-time systems. I think that’s the next step. If it isn’t the next step, then we’re going to have a mess. We’re going to have an absolute mess. We’re going to have a set of questions we can actually trust generative AI to answer. And a whole class of questions we’d better not ask because it thinks it knows the answer and it actually doesn’t. Who’s at work tomorrow? It’s going to think it knows. It’s got your team roster. You actually need that to come from the scheduling system. You’re managing a shop floor, you’re actually asking a question that has a very precise answer, not as you said Chris, it’s good at getting wide and close.
We don’t want wide and close in this case. I got to know who’s actually on the floor tomorrow. So we’re going to have to get AI to collaborate with systems in such a way that it knows when it’s got to invoke a real system, an actual backend live system as opposed to essentially extracted content from that, that is old the instant it lands on a disc. And of course, the very next step there is I’ve got an enterprise connected to this. I’ve just connected an enterprise system to a generative AI model so that now people can ask questions. There’s a whole bunch of questions they are not allowed to see the answer. So we’re going to have to sort out all of that stuff. So yeah, I want to know who’s on. Sure. It’s totally fine for shop floor managers to know who’s on the floor.
So what does that guy make? So Joe, he’s on, what does Joe make, how much does Joe make? You got access to the HR system for me buddy? What’s Joe make? What was his last performance review like? These are obviously inappropriate questions, so the trust is about thresholds. It’s about, what does it even mean to trust it? Because we have to trust it not to answer questions that is not appropriate to answer. We can’t just give it a boatload of content that was actually in public domain, which therefore makes it not its fault if it actually tells you. It’s not open AI’s fault if public content actually comes up in an answer to you. But it is entirely your organization’s burden not to answer a significant number of questions that your own people or customers may ask.
So we’ve got to do something much more interesting, and I haven’t even brought up the fact that humans will have to be involved not in vetting answers, but in actually constructing the answer. Is it feasible for me to take off tomorrow? Well a generative AI model is going to say, of course you just don’t show up for work.
Chris: Well, it might actually read the manual.
John: Which will say ask your manager. So what you just did is invoked a workflow that’s going to require the elegant orchestration of humans in addition to we’ve already established systems. And the enterprise data policies of those systems in order to make all of this work. So, man, I love the fact that we’re all talking about projects and the enterprise. We’re going to run use ChatGPT, but we’re literally like taking a whole box of fire, you know Black Cat firecrackers. Setting a fuse and tossing it in there and watching it all go. There are solutions to all of these problems. In fact they’re a great solution. We think we are one, but these must be thought through, right?
Chris: Yeah, I do think that there are certain things, if I ask it, it may tell me every employee gets 40 hours of vacation and 40 hours of sick leave, which would be accurate. But like you said, can I take off as actually implying that it understands the enterprise systems that knows how much vacation I’ve taken, do I need a manager approval on that? So I think it’s an interesting concept because I think our models will have kind of two facets. Some things are, we’re too lazy to read the policies and procedures and they’re static and we trained them. And on the other side, they do have to operate, it interacts with the operational systems. Like they’re going to have to figure out how to look up real-time data. Constantly updating.
John: Yes invoke systems and invoke people. Because you know, that policy Chris, everybody’s policy is yes you have, and of course the number of weeks varies by employee and type and how long you’ve been a company and whether you just start, all of that stuff, all of that becomes, those are all API calls. Even though everybody gets 40 hours a week is obviously not accurate at any interesting size company, but as soon as you ask a generative AI model, can I have off tomorrow? It’s only answer that is accurate is I don’t know, but it probably thinks it knows.
Chris: Oh yeah. I literally remember being at a large corporation and your salary band was in the inside of like, when you’re on outlook and looked up an employee, they’re an I, they’re a J, they’re a K, and the CEO was a Z. We’re like, so what’s the range for a Z? Of course the first thing it answers. So if AI could answer that, boy, would that be a problem?
John: My first question to Bard, what is band Z? Yes, there, you nailed it, Chris. So we are on the cusp of what is a fantastic opportunity to drive enormous productivity and to give people access to capabilities and information. All of that is great, but trust is actually, is such a, it may not even be the right word for it, because we’ve always been working under the assumption that we are getting mostly accurate but somewhat inaccurate information at times. And that we always have to vet in context all the stuff we’ve said. Where we are going now is we’ve got to set really clear guidance. Some things always have to go refresh a backend system to see some things not as often. Some things are actually people questions.
Can I have off tomorrow, must be a people question, if I’m on a shop floor or if I’m a delivery driver or whatever because someone’s going to have to cover my shift. And that is not going to be just a systematic answer. It’s going to be, let me go find someone who can cover your shift and get right back to you. That would be the best possible answer being compassionate. If I really do need off, let me send a message to as many people in your role who are already not on the schedule to see if one of them can cover your shift. And as soon as I get a confirmation on that, I’m going to inform a whole bunch of people who would obviously need to know this because they want to know who’s going to actually pick up the goods and get deliveries going and get back to you, Mr. Employee who needs off with that solution.
That’s a whole interesting outcome initiated from, of course, a natural conversational chat. By the way it’s obviously a great Krista use case. But generative AI being an entry point and an exit point and even facilitates along the way point. When one of those employees gets that, hey, can you cover for John tomorrow? The immediate response is, what’s the rest of my schedule this week? You can’t say, does not compute I needed a yes or a no, dude. It’s real humans respond to questions often with questions, we call these dynamic sidebars. Well, what’s the rest of my schedule this week? And that again, is here goes a real-time lookup. But with that context, I now can trust, I can say yes. And with that context, the guy who actually gets, is needing off, let’s say me comes back with manager was informed, policy’s fine, manager’s informed, Chris is confirmed. I trust that it’s actually fine. I’ve done my part. So I think we’re in a fantastic opportunity to create this kind of solution for customers, but it is clearly a component, not the solution.
Scott: I just hope that the system wouldn’t update the hours taken because I would just ask every day for the day off. Because you would have to trust whether or not it actually closed that whole loop because maybe I become Band Z and I just ask off every day and then till someone catches on, right?
John: You know there’s always someone trying to game a system. And speaking of HR in a total digression talking about sidebars, was talking to somebody very early on when we were building the product. He said, when I would go into the HR system and ask off, I got the ugly looks like a mainframe and was a green screen. And I thought, how many hours are you looking to take off of your PTO balance? He did minus eight thinking that would be subtracting eight but minus eight is plus eight. So he ended up going from 40 to 48 hours of available PTO and got off that day. So he promised he only did that a few times. But that’s a digression that it’s about the garbage in and garbage out problem and how we’re expecting our humans to fully understand how systems think and work. But that was a classic.
So yeah, how do we trust even the HR system to input a correct number of hours? We all live in this world. AI will learn to get there. We’ll need to understand how some questions are real time in nature. Some are workflow in nature. Some are completely inappropriate for them to answer or for them to know the answer. All of that is a fabric that has to be built around this tech.
Scott: Hypothetically, John, if I’m putting something like this inside an organization and I’m hooking up a generative AI so I can have conversations with systems. What type of, and I’m going to call it like a sales process or training. Like how do I talk to my internal employees to trust this process when they have been trained on the internet bias. Of all the horror stories, like, hey, we’ve done this. Now you can talk to the different HR systems to take off and communicate with your manager. Like that seems like it would be a whole sales job, internal sales job, right?
John: Well, you might have to just recreate the whole trust solution and planning that Google, open AI and the other guys are already going through where they’re going to give you more context. So if step five of that requesting leave process back to you, Scott, was, okay, I have performed this, your HR solution is Workday and I have removed those hour or pending, right? Those are now pending medical leave hours and your manager whose name is John has been informed and blah blah. As soon as you don’t say, just sure have a great time. As soon as your answer has enough context for you to really be, it sounded like real stuff happened back there because it actually knew where to go to log the leave request, it actually knew the manager that it should be informed or asked depending on the policy, whatever it is.
And yeah, I think I can get there, I think that just actually happened. You at least have also the audit, again, not currently in the online versions of where they we’re seeing and playing with, but in the enterprise deployed version of a tech like this, you obviously also have the audit trail of, hey, I had a conversation with this thing and it’s all being stored somewhere. It’s even in my chat history. So you don’t give me this, allow me to ask that question and then tell me that I can’t trust its answer. So I would imagine this is the way you’ve got to go. And of course there will be an evolutionary or maybe a certain wave of swell. It’ll start like, it’d probably start with fury of activity of dudes like Scott trying to take off every day and seeing what happens.
But after that little discovery thing how well where the guardrails put up, there’s probably a pretty good ramp of utilization where it’s like, wait, well why don’t I try it this way? Why wouldn’t I? Wait, why don’t we do everything this way? Why do I have any of this stuff? Why am I not just? So of course that’s been our philosophy and our position, the way the world work from the beginning. So I hope it does, and I think that this is a good step.
Scott: And I hope that people would, especially, we’re talking about this time off and vacation time, I think that’s an easy example to think about. And then if everybody interacts with this, they’ll understand their job enough. Like, wait a minute, I can apply something like this to what I have to do because I’m just reading a system and making a decision anyway. Chris, if that happens and we’re about out of time, so I’ll ask you to sum it up, like if I’m using this internally, what type of things do you think that people would think of, like say for instance I’m like a site reliability engineer at a software company or something. Like, what kind of things could I do that would help me?
Chris: I think we’re going to find huge categories in every line of business. So like we mentioned, HR has got stuff, we’ve got things like in cybersecurity, like the ability to actually ask them about threats in that. So no one wants to go look up a threat on the internet and say, is this a well known thread on email? And those are things that we can look up, some historical stuff and then we can look up on APIs both and customer service, think accounts payable, accounts receivable, all those emails they get, where they literally are answering the same questions over and over. If it’s a policy question, that’s something that an FAQ would be waived in. But then you’ve got the API, so look up what’s the status of a specific order for last week, things like that. So I think we’re going to find a blend of FAQ type things and API things in every part of our business.
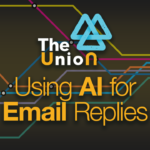
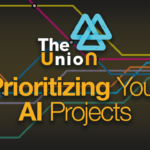