AI-driven business processes are becoming increasingly popular, as they can increase business velocity. Using AI to respond to email and omnichannel messages allows businesses to respond quickly and efficiently while providing a higher level of customer service. However, AI by itself is not always the answer since there are two paths you can take with this technology – task-based automation and conversation-based automation.
Task-based automation involves creating simple rules for computers to follow when answering emails, like sending out standardized responses or performing basic administrative tasks. This solution provides an efficient way to provide basic customer support but doesn’t provide much benefit beyond that. Conversation-based automation, on the other hand, uses natural language processing (NLP) and machine learning (ML) algorithms to understand the context and intent of customer emails to provide an appropriate response without the need for a person.
To train AI-based resolution systems to understand your company-specific language and context, you need to provide the appropriate training data. This can include providing sample emails from your customers so that machine learning algorithms can learn how to classify them according to different categories. Additionally, you can also use feedback loops so that your employees can manually reply or verify the machine-generated reply before it’s sent to the customer.
Using AI to reply to emails provides you with several benefits, like immediate response times, greater customer satisfaction, and cost savings. For companies looking to implement AI-based solutions for their customer support needs, understanding how to properly train systems is key to achieving maximum benefits. As AI technology continues to evolve, we will see even more improvements over time. In any case, using AI to reply to email is an important tool for businesses that are looking to stay competitive in today’s marketplace.
Links and Resources
Speakers
Transcription
Scott: The whole chat GPT thing changed the way people are looking at how software or how AI can answer a question. But that’s more conversational between like me and a machine. But what it did is, I think it changed everyone’s view of what’s possible. So previously we’d covered, how chat GPT elevated our expectations, and by our, I mean, everybody. So this thing, it’s cool, but this functionality has been out there. People just didn’t really see it. So the chat GPT phenomenon has really helped elevate the expectation that we have. And now people are contacting us about email. Like, hey, I’ve got all these incoming emails from customers or from partners. I can’t get to them fast enough. They come in faster than I can respond to this. Can AI help me answer these emails?
Chris: Scott you’re right. I’ve talked to several customers, like, we don’t get a couple emails. We get 500, 1000, 3000 emails a day. Like it is something we need computers to scale to help us solve the problem. Because it’s, not like, oh, I got three yesterday. They’re literally saying it’s 500 to 1000 and more a day. It’s huge.
Scott: Yeah. It’s a lot. And it’s all across the board. And it’s nothing more than someone reading the email figuring out where the answer would be, going to a system, looking up the answer, typing an email, and then replying. But you can’t do that 3000 times.
Chris: I think you just identified the problem. It’s like looking up the answer because we know chat GPT knows what’s on the internet, but you said, looking at the answer that means, well, in my enterprise and my ERP and my customers CRM, in my proprietary systems, looking at the answer is the problem. That’s why humans are doing it.
Sam: And it’s so dissatisfying. It really is because the way that people have been scaling has been form answers. You can sell it. It’s just oh, it’s kind of related, your question is kind of related to this. I’m going to give you this standard prefab answer, which really just disheartens the person that just spent a lot of money with you.
Scott: Yeah. Because they probably already read the answer on your FAQ doc. Like, if you have a help page, hey where is my, or how soon can I? You’re always like waiting to receive something, but that’s like the next level of information. If I order something, where’s my whatever it is, right? If you get a tracking number, like I can look that up in another system. But how would I converse via email or maybe even omnichannel like WhatsApp is popular. I guess I’ve never WhatsApp anybody for a customer service issue, but I guess it’s possible.
Sam: You know, I found that somebody did that to me in Europe. I went to Europe last summer and sent an email and the reply came in WhatsApp and that was the first time I actually used WhatsApp. It was on my phone and I had one of those little badges on there and I opened it up. I’m like, wow, look at that. They’re responding to me in WhatsApp.
Scott: Yeah. So I think with what this can do, so Sam, like, I mean, we’re talking about these customer service issues and I think the contact center, right? If you have a bunch of people trying to serve customers coming in through the internet, what’s something that they should look at, like take a next step? Because obviously this can be done. Like how would they do that?
Sam: I think a lot of people struggle with that first step Scott, and that first step is because when you look at the tools, I mean, you mentioned chat GPT earlier, but that’s all trained off Wikipedia and other sources like that. It is not specific to my organization. So you need to find something that has that type of flexibility and that type of capability. You have to think about your end user and you have to be able to make it simple enough but robust enough that it can use your own history, not the history of the internet or the history of Wikipedia, but your own internal knowledge, your own knowledge base. Be personal and then also be extensible. So I would imagine that 80% of the emails coming into most companies are going to ask questions that are easily solved.
And then it’s really only the 20% that have to do that, start that interaction in a nice personal way that doesn’t really upset somebody. So that when it finally gets to the new person that is going to actually respond to your email, that they can actually do something and help and see that the previous interactions have been helpful. So I tend to think in this model we have a lot of customer service agents, but also if you look at, I mean, I think that some of the worst people at this are the interns that answer emails for your Congress people. They’re absolutely terrible at this.
You ask them a question three months later you get an email back and it’s about a topic you didn’t even ask about. And bless their hearts, they’re trying to do something, but they don’t have the knowledge to do it. The knowledge exists. If we can harness that knowledge through AI like you were talking, reply back in a nice actionable way, that’s a win.
Scott: Yeah. So you said next person, right? So that’s interesting because like, there’s high turnover in those organizations and that is probably like the least trained employee. And then when the person that knows it all, when they leave, like it’s just havoc.
Sam: The institutional knowledge is gone.
Chris: All that tribal knowledge and I think the worst experience I’ve had is I knew some email addresses at a company and it emailed a question and it probably went to the wrong email address. So think I asset and accounts receivable question to someone’s accounts payable. Well, they just closed the ticket because they didn’t actually have the ability to change it to a different part of the organization. So the way a lot of these emails are is a company may have 20 emails and they’re specific to a department, specific to a role. So if you don’t send it to the right person, it’s not like internally they know how to transfer it and send it to someone else. They just close it as done, wrong department.
Scott: Yeah. And I think that’s evident when the companies email you, right? Because sometimes you get an email from someone that you buy services from and I think I get these from like T-Mobile or something, and it says like, hey, do not reply like this email box is not even monitored. I’m like, wait a minute. Like, you’re going to email me and if I reply, you can’t even answer me. Like, that is ridiculous.
Sam: You must take action now. Well, I got a question. No questions allowed.
Scott: Here’s your offer. I think it is T-Mobile. I think if you want to do this and you’re watching or listening, go to your inbox and just type in, do not reply as in your search inbox field, and you’ll see what I’m talking about. You’ll see all these emails that you get sent that people don’t even want to hear from you.
Chris: But wait, Scott, we can solve this. We have the technology.
Scott: So I mean, if it’s possible, Chris, like how does it work? What do we do?
Chris: Yeah, so this is not the $6 million man. This is actually technology that exists. So AI, artificial intelligence is literally a computer thinking and acting like a person. And it’s not magic, it’s math, it’s hardcore math algorithms. And we do certain things. We have a machine learning module which knows how to classify a request. So it like reads a paragraph or a couple paragraphs and says, oh, this is a question about an order status. This is a question about a delivery status. This is a dispute. So with machine learning under the big picture of AI, we actually have the concept of classification. So that’s the first thing we do. The second thing we would do, and this has been around a very long time sentiment analysis. So even10 years ago when you called a company and you were getting aggravated on the phone, phone telephony systems were converting that to speech, to text and then looking at sentiment, are they neutral? Is this positive or negative? It’s just a [10:06 inaudible] things.
So when we get an email, we want to do the same thing because if we see that it’s negative, we need to bump the priority. So if that means respond faster, we do need to escalate to a person. We can do that. And that actually does help solve part of this problem. And then the neat thing is, and this is a little bit newer, in the computer world, we call it entity identification, which is a really fancy word for, what are they asking for? Are they asking for.
Scott: That’s two words though.
Chris: Oh, yeah, I guess so entities is the one word. Entities, and can we identify them? So it’s like, is there a date on here we need to look up. Is there an order number inside of here we need to look up, is there something about a purchase order or an invoice or something like that? Or do we reverse back the email address? So the idea is when they ask the question, it’s like, when will my shipment for PO or invoice 57 show up or order number 2456 show up, that’s where we are looking for the data. And then the cool thing is we can actually take action on that. Like, we could actually write an automation to look at the order number and reply back with an answer. So there’s the whole concept of let’s classify it, let’s figure out the sentiment. Are they happy?
And if it’s something like a dispute that’s going to go to a human workflow, if it’s something that we can take an action with automation, like order statuses, is this in stock? What’s my shipping? Blah, blah, blah. Those things, we can actually identify information in there to answer the question, and I think the place that’s going to go is going to get more interesting. So there’s a little dabbling that we see in the consumer realm, but there’s a lot of computer vision out there. So how cool would it be if you emailed the company for your sprinkler system and say, I need the manual for my sprinkler control box, and instead of turning it upside down, identifying the model number, if you just take a picture of it and email it, and then computer vision says, oh, this is model A 45, and they send you the manual, or they send you instructions on how to change the time because daylight saving time is coming. Can we actually get very actionable and answer the question? And in today world, those technologies, they’re not perfect, but they’re there and they’re getting much better. And we can use them today.
Sam: I think part of that problem, Chris, is that some of those technologies are there, but they’re being piecemealed together. And that piecemeal provides glimpses of a good experience, but overall it’s not a good experience. And being able to coordinate and orchestrate all this into one process is the last piece that a lot of companies haven’t taken, they haven’t used their internal knowledge, they haven’t used the cool technologies like computer vision to simplify the experience and to reduce the number of back and forths that have to go on. So you can, which all those things when you don’t put it together prolong the experience versus making it so that your customer can get back to doing what it is that they were hoping to do.
Chris: Yeah. Like a lot of technologies you have to orchestrate chat GBT would actually be really good to give you an FAQ from your enterprise, but then you do need something from sentiment and then something that does entity identifications and computer vision, and then some type of orchestrated workflow and automation across the back of it. So there is a concept of we are bringing together multiple technologies and different people are good at it, but when we bring them together, we can solve this problem.
Scott: Yeah. I mean that’s a problem indicative to how companies are set up. So they budget and they schedule jobs in departmental silos. So I think this is the rise of the chief digital officer that kind of looks at digital processes across a company, across all the different silos. Because if you’re inside the silo, you don’t see the problem. Like, okay, hey, we want to be able for people to send us pictures of their sprinkler system, control box, and then just automatically reply back to them with the manual or the question. But in the workflow process, that could be a much larger issue. Like, hey, why do I need a manual? Well, maybe I did a home edition or I had an extreme failure, I need a maintenance call or whatever the other situation is. Like, that person doesn’t see it, but the digital officer that sees the customer journey, I think would identify something. So I think those type of people are going to be really looking into this chat and AI functionality because they’re looking at the journey and the process which you guys were talking about versus just kind of a, hey, can I solve some small issue, right?
Sam: You stuck something in there, which I think if you looked at the broader context, you can really see what that problem is. And Scott, what you had said was something came in and there’s a broader issue and there’s another department that’s involved, and that’s the piece that you snuck in with, there’s another department involved. A lot of people have been trying to solve this with tools like RPA. RPA is okay for tasks, and those tasks generally live in one system, but when you try to cross systems and cross departments those tools really aren’t set up for that. So now the attempted automation to improve the experience is impossible, or even worse, has failed. And the end user either way has now delayed or somebody thinks that they’ve satisfied them, but they really haven’t and it’s really become quite awful. So being able to orchestrate across departments, across systems seamlessly is really a huge benefit for the customer, but will also unveil those hidden things that you were just talking about.
Chris: Yeah. So I think to overusing a slang from the US, don’t lose the forest for the trees. So the chief digital officer is responsible for the entire forest and all the trees through different departments so how does it work together?
Sam: How you take the information that’s on a single leaf and give it to somebody who’s in the warehouse, if you are sitting in a accounts payable.
Chris: Yeah. So I think this is something automation cannot solve. And then I think people will realize that there are two paths, and some people may have a bad test, like you mentioned they’ve tried this with task based automation, it doesn’t work. But it’s completely legit to say if you can solve 80% of the questions through a computer replying back through conversation, and then 20% actually get escalated to the person and you’ve ranked them by sentiment, you’ve actually have a big business win.
Sam: You Do. That’s why you take the do not reply on there because they can’t today reply to all of those emails at all, they just can’t do it. But if you can solve 80% and now it can reply just to the 20%.
Scott: Yeah that sounds good. So Chris as we wrap up, I think people will wonder how do I train the answers. So if I can answer 80%, like how do I, okay, I’m sold on the chat GPT functionality except, we talked about that was trained on Wikipedia, I needed to be trained on my business and how I answer. Why don’t you give us a summary on how that would work and then we’ll close this session.
Chris: Okay, so there are two things we can do. You can actually train chat GPT on your company’s FAQs, on your company’s data so it can reply back and not give an answer off the internet. It can actually give you a local answer that you know about. So that’s actually within the technology. The other thing is when we train the machine learning for classification of emails, we actually want to take the emails from a specific company, because every company’s vernacular in the way they refer to things is a little bit different. So it’s literally an act of, okay, give us 10,000 of your emails and if you put them in the CRM and you classify them, let’s extract that report and we’ll train machine learning on that. If someone manually replied, we’ll classify the responses and train on that. So we can actually make an enterprise capable by taking the specifics about your company and chat GPT is capable.
Machine learning we want to, because even if it’s a sprinkler company, a pool company, a company that makes roofs, someone who does travel reservations. Those type of questions are different. Customer service is very broad. It could be cellphone, it could be cellular, it could be water, it could be electric, it could be almost anything. So we do want to get training data from your enterprise, and then machine learning will answer in the context of your enterprise. So we can do that with examples that you have.
Scott: Alright. I love it. Well, thanks guys, and thanks for talking with me about AI answering emails. I think it’s a good idea and everybody should do it. Alright. Until next time.
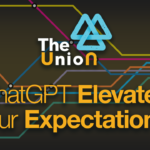
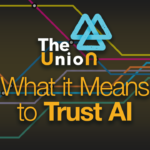