ChatGPT’s recent popularity is causing business leaders to ask for more AI apps. The demand inside enterprises is so high chief information officers say they cannot keep up since building, training, and rolling out AI models is so expensive and takes too much time.
Are business leaders focusing on the right problems?
Business leaders have seen generative AI and it has changed their expectations. Now that they have seen it, they are asking how to incorporate generative AI functionality like ChatGPT into the enterprise. However, the sudden demand has flooded the CIO’s organization with very challenging project requests. They cannot say “yes” to all of the requests.
Not every AI project requires a data scientist
Business leaders seek AI to help them make decisions and they often look for the highest potential value project. This makes perfect sense. They want the biggest possible gains when deploying their resources. But, at what costs?
Now, they want AI and they want it fast. But, CIOs can’t fulfill all of the requests. Many of the requests are resource intensive and take a long time. Some AI projects require enormous data gathering and cleansing steps and then need data scientists to spend many months training models to identify features in those datasets. This process can take up to two years to complete, requires constant monitoring and maintenance, and then requires an intuitive user interface for the model to become useful.
These projects seem like moon shots.
Are there simpler ways to deliver AI?
Yes.
If the highest costs of custom AI projects are data scientists and there aren’t enough of them for all of the projects, then it behooves you to optimize around these constraints. If you focused on the hardest problems to solve but lack sufficient resources and time to solve the complex problems your project will fail.
You need to find ways to deliver AI that don’t require custom machine learning (ML) models and expensive data scientists.
There is more AI value in higher-frequency processes
You can derive more value from using AI in higher-frequency transactions than betting on your moon shots. By applying machine learning to smaller processes in a company, which happen hundreds and thousands of times a day and are worth a small amount each time, the cumulative value can add up quickly. While these transactions may not be as valuable as larger transactions on a unit basis, there are more of them and they are run against every customer, presenting a significant opportunity for AI to be leveraged. It is not practical to put a data scientist on all of these higher-frequency transactions, so a different approach is needed. Machine learning can be used to address the 80% of transactions that are lower in value and higher in frequency, while still allowing data scientists to build custom models for the larger transactions. By combining these two approaches, companies can maximize the value derived from AI.
How to prioritize AI projects
Project managers sometimes overlook the potential of simpler AI projects because they are focused on building a model that will give accurate answers right from the start. However, it’s important to remember that AI can also be assistive in a process, and we can break down the deployment of AI into smaller, simpler projects. By presenting users with suggested answers and allowing them to confirm or change them, we can improve the accuracy of the model over time. This also helps with model drift, which is a big problem when things change in the business environment. It’s not just about building the model, but also about continuous monitoring to keep it up to date. When we include people in the process, they can provide feedback in real-time, and the model can be regenerated and tested for accuracy. It’s a mindset shift from creating the model to figuring out how to employ it iteratively and then continuously monitoring and improving it with user feedback.
Links and Resources
Speakers
Transcription
Scott King: Hello, I’m Scott King, and that’s Chris Kraus. Thank you for joining us today. We’re going to discuss a recent Wall Street Journal article. I found this article interesting a couple of weeks ago. The title is “Pressure Mounts on CIOs to Build More AI Apps and Faster.” We previously discussed how GPT has elevated expectations. Now business leaders are asking CIOs for more AI and to deliver it quickly. The article says CIOs can’t respond fast enough. I think maybe they’re focusing too much on the top 20% of AI and machine learning projects. What are your thoughts on that?
Chris Kraus: In my interactions with customers and prospects, I’m seeing the same thing. People are asking how they can implement ChatGPT in their enterprises. There’s a classic approach to AI, which involves data scientists working on very hard problems for months, but the catch-22 is that the hardest problems can take years to build a model for and require ongoing monitoring. So, we need to consider whether we’re focusing on the right problems or if there are other processes in the organization we can work with.
Scott King: The article suggests that the bottom 80% of processes have greater collective value than the top 20%. There must be many processes where AI and machine learning can assist in decision-making, rather than focusing on complex actuarial tables or risk management. The article states that the value in the bottom 80% is probably greater than in the top 20%.
Chris Kraus: Yes, if you think about it, everyone uses the 80-20 rule. If you have many smaller processes in a company with good velocity, even though the value may be $10 or $20 versus $10,000 per transaction, there are more transactions and more of them. We need to think about a different way to address the 80%, using machine learning and putting it in the hands of users.
Scott King: One of the CIOs interviewed in the article mentioned spending several years and $20-30 million on an AI project. The cost of data scientist salaries was the biggest factor. It’s interesting how on one hand, it’s all about solving the data problem, and on the other hand, making AI intuitive and useful is a challenge.
Chris Kraus: Absolutely, those are two different disciplines. Data scientists are skilled in working with numbers and tables, but they aren’t the right people to design an intuitive user experience. There’s a huge challenge in transitioning from building a model to operationalizing it and making it easy for users to interact with.
Scott King: Over 90% of AI models don’t go into production because they can’t be deployed. This is where the phenomenon of generative AI and conversational user interfaces comes in.
Chris Kraus: Yes, chat GPT has raised expectations for conversational AI. People want to interact with AI in a more conversational manner, rather than through complicated forms.
Scott King: So, regarding the cost perspective, can you discuss the cost of implementing AI and how companies might be misjudging what’s required for simpler use cases?
Chris Kraus: I understand that project management teams might say they don’t have enough resources for a special project for every model. These models need an interface for data input, system data, and a user interface. Project managers may argue that they can’t add 150 more applications to their backlog because they don’t have the velocity to do so. Instead, they need to deploy AI iteratively within a single platform, making it easy for users to ask questions and have the platform provide responses and take actions. It should be one project with a good orchestration engine to deploy it.
Scott King: That makes sense. Everyone needs a process because you can’t train people to do everything, especially when apps and infrastructure change. There’s a standard operating procedure for everything.
Chris Kraus: Exactly.
Scott King: Everyone has all these run books, help docs, and intranets. Just make the software do it. I didn’t read the manual to install a smart thermostat; it just did it for me.
Chris Kraus: Yes, and ChatGPT, with its conversational approach, raises expectations. People will want to interact with AI in a conversational manner instead of using an unattractive form or navigating through multiple steps.
Scott King: Everyone knows how to have a conversation, so we might as well use that approach. They want to see how AI works within their business and operationalize it. Focus on the easier tasks and learn along the way.
Chris Kraus: Part of the challenge is that when you build an AI model, you want it to give accurate answers, but it might not be accurate from day one. By deploying AI in an assisted manner, users can learn and trust the model. Presenting the AI’s suggested answer and confidence level allows users to provide feedback that helps fine-tune the model.
When dealing with simpler examples, users can easily determine if the AI’s suggestions make sense. Including users in the process helps address model drift and changing business environments. By giving real-time feedback, users can help regenerate and test the model for accuracy. It’s a mindset that needs to be seamless and continuous across the realm of AI implementation.
Scott King: That makes sense to me. Hopefully, others will catch on as well. Chris, I appreciate your time. Thanks for discussing the article with me. Until next time.
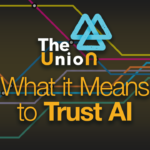